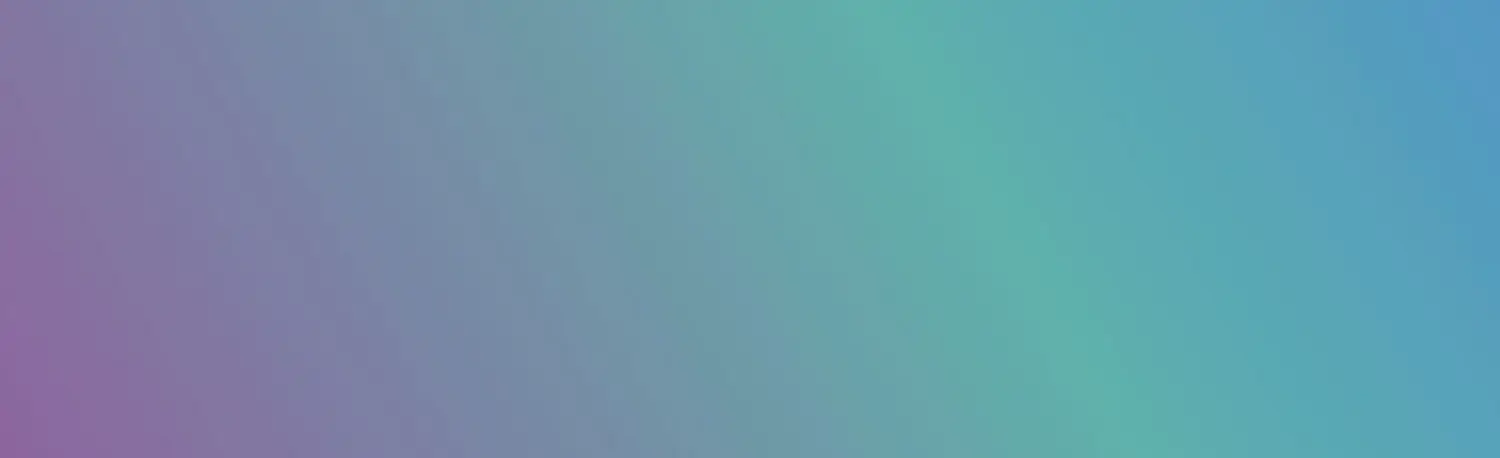
On decision-making in HR – in conversation with Olivier Sibony
For our joint study on AI, “Leadership in the Age of Technologically Assisted Decision-Making”, Prof. Dr. Miriam Meckel, Dr. Léa Steinacker, Fabian Kienbaum and Lukas M. Fastenroth interviewed several experts on Artificial Intelligence. One of them is Prof. Dr. Olivier Sibony, to whom we talked about morals and decisions. He provided interesting examples concerning the future nature of decision-making in the context of HR, which we would like to share with you.
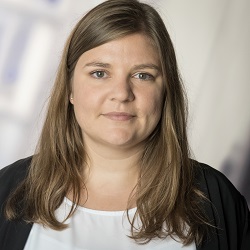
Kerstin Wandt
1. Olivier, could you imagine us reaching a point where a manager is held accountable for not considering a machine calculation or recommendation in his decision?
That’s already the case today. One very basic example: if you are a credit officer and there’s a number of automatically calculated risk ratios that inform your decision, and you choose to override those ratios and take a risk you shouldn’t have taken in the credit committee, you’ll be accountable for that decision. Whenever there’s an AI or algorithm-assisted decision, you’re accountable for that decision. And the problem is that it creates an asymmetric incentive. It leads you to be more risk-averse – and there are several arguments against risk aversion. Suppose you’re a judge who is making a bail decision. The machine tells you this person is scored high by the algorithm, but you actually trust them. It’s a big risk for you as the judge, because if the person is freed and reaffirms or fails to show up for the trial, you’ll look like a fool for overriding the cautious algorithm.
On the other hand: if the algorithm says we shouldn’t send this person to jail and you send them to jail, no one will be able to see the mistake you’ve made. Because you’re more risk-averse than the machine. So, the trade-off is always asymmetrical. It’s the same for credit committees. If you grant credit that you were told by the machine not to grant, you are taking a risk. Sometimes it might turn out OK. But sometimes it’ll be wrong.
And the times when you’ll be held accountable are the times which will never be studied properly because people only concentrate on the spectacular mistakes. So, this idea of teaming up with machines to make decisions is always a challenge because of the asymmetric incentives.
2. But what if the machine makes better decisions based on poor data? Take HR, for example: You’re trying to decide between me and Peter or Bob – obviously, Amazon had this very problem. Their AI predicted that men would do very well at Amazon, and it did so brilliantly. The mistake was made by humans in the past: it was the fact that they didn’t hire women. This is, I think, not even a technical question. What do we do about all this?
It’s a very technical question. It’s the problem of biases in algorithms and data. As you point out, the problem lies in biases in the data that algorithms are trained on. This isn’t contrary to what a lot of people are saying.
The problem is the bias of algorithms. Because algorithms are designed by 35-year-old white males in Silicon Valley, and therefore cannot account for the experiences of people of different genders, races, or ages.
When you train algorithms on biased data, those algorithms will be biased. That’s guaranteed. Where there’s data, it will be biased. That’s guaranteed, too. Therefore, all algorithms are biased. That’s certain. The question is, what do we do with this fact? Now it gets really interesting, because the beauty of an algorithm – unlike a human judge – is that you can actually fix it.
Take the example of Amazon and its biased HR algorithm. They made the decision to trash it because of the scandal it created. That’s stupid. It would’ve been very easy to fix that algorithm. It’s much harder to fix the humans who are making those biased decisions. What the algorithm is actually telling you is, “Hey, Amazon, I’ve got news for you: your humans are biased. The data we’re looking at is telling us that you’ve been making biased decisions for a long time, so you should do something about that.” Reacting by saying “Let’s trash the algorithm and go back to the old system” is the worst possible decision, because you go back to a situation which is biased.
Why does that situation not strike us as unacceptable but the algorithm does? Because again, we hate the algorithm and we like ourselves, as human beings. It’s also more visible in an algorithm than it is in humans. It’s more visible for two reasons: One is psychological and the other is technical. The psychological reason is that the algorithm is free from noise. Because the algorithm is free from noise, the sexist algorithm is going to hire men every time, basically. Whereas even the most sexist recruiter is occasionally going to hire a woman. He’s going to have a day when he feels somewhat inclined to hire a woman. Some woman is going to remind him of his sister or something like that. And despite being horribly sexist, he’s occasionally going to hire a woman. He’s not going to be as disciplined or as rigorous in being a sexist as the algorithm that’s trained to mimic him.
The algorithm will look at the past decisions that have been made and say, “Yeah, it looks like you’re doing sexist things. We’re only going to hire men.” Then the algorithm is going to reliably do that in full, consistently, in a noise-free way. So, it shows more when the algorithm is biased. The algorithm is just as biased on average, but it’s more reliably biased in its decisions. Plus, of course, you can test the algorithm on a million cases and actually quantify the fact that it’s biased. And assess why it’s biased, which you can never do to yourself or the recruiter who only sees 10 people a week because there’s a limit to how many they can see. And the same people cannot be seen by another hundred recruiters to compare their decisions with his.
So, the algorithm would actually be a very good solution to this problem, if only they’d fix it. This is the problem. This is the challenge. The reason the algorithm is making those biased decisions, is because those decisions match what the algorithm was told you wanted. Telling the algorithm what you want forces you to clarify your objectives in a way you’ve never done before.
Take the example of male judges. We know we have an algorithm that can result in either a lot fewer people in jail with the same level of crime, or a lot less crime with the same number of people in jail. We now have to choose between these two outcomes. What does society want? Does it want fewer people in jail and just as much crime, or does it want to minimize the number of crimes by putting just as many people in jail as today? Or more, in any case. Do we want even less crime with more people in jail? By the way, we can also fine-tune this and say, “do we want racial equality in the number of people we’re putting in jail at the risk of damaging our objective for the number of crimes committed?” It forces us to make trade-offs between different objectives that are very uncomfortable to make.
Amazon, do you want to lower what you regard as the quality of your hires based on your past decisions – not the actual quality, which is very difficult to measure – in the interest of diversity? To what extent would you want to lower that quality? These are very difficult trade-offs to make. And as soon as you make them, you run into all kinds of problems, including legal ones.
3. Considering everything you’ve just pointed out, which is very interesting, what core competencies should a future leader have to deal with these challenges?
Rationality. A willingness to make rational decisions in the face of emotional reactions. An understanding of risk and insurgency. And the willing-ness to take rational risks and reject irrational ones. And a sufficient understanding of how technology works to manage the reactions and fears of people dealing with technology, which are going to remain present for a long time.
Thank you very much for your time!
About our interview partner
Olivier Sibony is a professor, author and advisor specializing in the quality of strategic thinking and the design of decision processes. Olivier’s latest book, “Noise: A Flaw in Human Judgment”, co-authored with Daniel Kahneman and Cass R. Sunstein, has appeared on multiple bestseller lists worldwide, including the New York Times list. Find out more about Olivier here.
You can request the study report for free:
Do you have any questions?
Feel free to contact us anytime: studien@kienbaum.de.