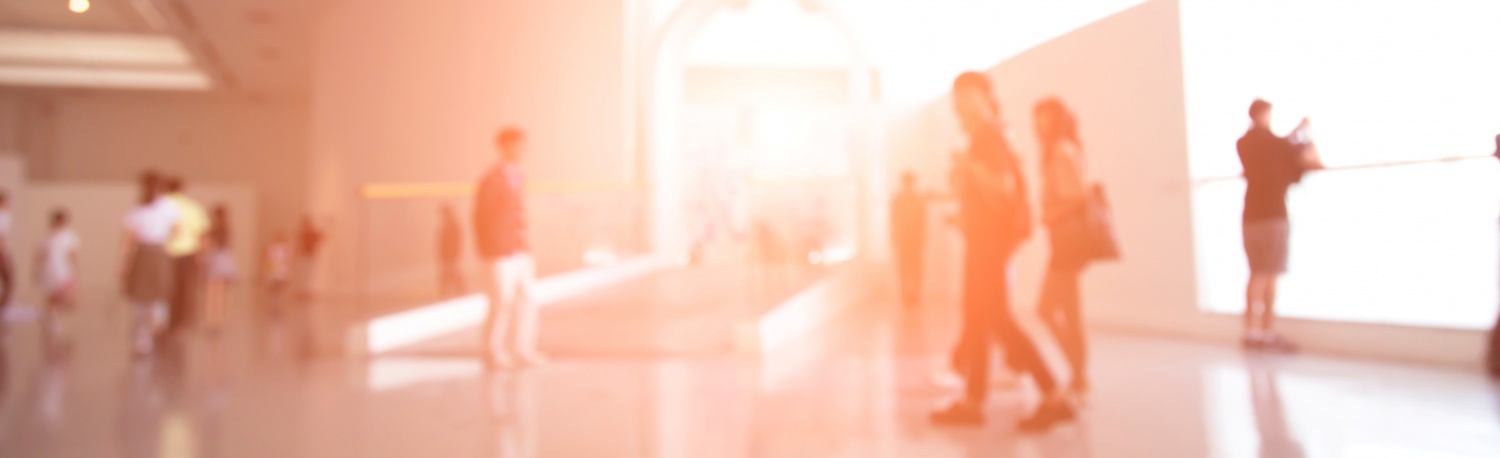
Organizational Network Analysis – Case Study
Even though Organizational Network Analysis (ONA) has been named one of the biggest trends in people analytics, the amount of use cases available is surprisingly low. Used right, ONA can be a scientific method to measure previously hidden organizational KPIs and at best, provide a completely new perspective on organizations. At Kienbaum we …
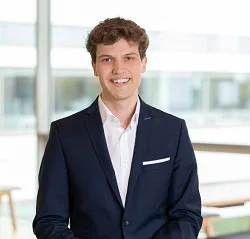
Yannik Leusch
Even though Organizational Network Analysis (ONA) has been named one of the biggest trends in people analytics, the amount of use cases available is surprisingly low. Used right, ONA can be a scientific method to measure previously hidden organizational KPIs and at best, provide a completely new perspective on organizations. At Kienbaum we have used ONA to understand and visualize the effect of our very own organizational transformation. We aimed to break silos, empower collaboration and facilitate the exchange of knowledge. ONA did not only enable us to track our success but also provided us valuable information in continuing our transformation. We’d like to share it.
What is ONA?
Organizational transformations are hard to plan and execute and even harder to measure in their success. Advances in people analytics and data science have however enabled us to leverage a tool, whose original method and theories have been around for decades: Organizational Network Analysis (ONA).
Social or Organizational Network Analysis is a method which depicts organizations as a group of individuals that are connected to each other, based on interactions and relations in everyday life. The resulting network can be mathematically analyzed for different characteristics, such as the level of interconnectedness within the network or the existence of isolated groups or silos. So other than an organizational chart, a network visualizes the organization based on real behavior.
Data gathering
Organizational networks are created based on data about some sort of interaction, relations or behavior. Typically this can be anonymized mail traffic, mutual calendar entries or skype calls. In our analysis we have opted for a less intrusive and privacy preserving source of data. As a consultancy our daily work is organized in projects that we are staffed on for a given period of time. With a little bit of data crunching we pulled a list from our CRM, containing the information with which colleagues every consultant has been working on the same project over the past two years. Even though it doesn’t capture informal interaction, project data is a good start for operationalizing collaboration within the organization.
Data analysis
After preparing the data and organizing it into nodes (employees) and edges (mutual projects) we began our data analysis with analyzing the topology of the whole network and found no surprise: the organization is formed out of two big sub-networks that constitute our two core businesses: management consulting and leadership advisory.
The coloring of the nodes in the screenshot of the network labels different competence centers. We identified strong collaboration between specific competence centers but also potential for more collaboration and cross staffing between competence centers that could benefit from each other’s expertise. We identified key players and connectors that bind together different sections of the company and small groups that are very much focused on their own projects. This is not necessarily good or bad, in the first step it is a mere observation based on data whose interpretation heavily depends on its qualitative context.
Our original goal was the analysis of our organizational transformation – the result of the transformation on our project staffing and collaboration. We wanted to break silos, enhance collaboration and facilitate the exchange of knowledge to better serve our clients with the broad expertise in the firm. When it comes to organizational network analysis, the biggest challenge for people analytics so far was to come up with ways to interpret and translate these network metrics into business relevant KPIs.
To assess the changes in the organization we split the data set in half and analyzed the years 2017 and 2018 separately. By comparing two states of the same network changes become apparent and network metrics, such as the density of the number of distinct clusters can be confronted and interpreted.
In the comparison of 2017 and 2018 we looked at the business units that were affected most by our transformation – the management consulting division. While in 2017 the network could be segregated into 8 clusters, in 2018 the network has substantially condensed, with the space between different clusters becoming smaller, reducing the amount of clusters to only 5.
Next we analyzed the level of collaboration between different centers of competence. As there is no standardized metric in graph theory that describes collaboration, we operationalized the cross functional collaboration as the ratio of connections between employees of different units and the total amount of connections. Thus this collaboration score provides an indication of how strongly the organizational structure limits collaboration.
The collaboration score can range from 0 (only collaboration within business units) to 1 (only collaboration across business units). Between 2017 and 2018 our cross functional collaboration has increased from .16 to .23.
So what?
Organizational Network Analysis is a rather descriptive than predictive or inferential method in people analytics and it doesn’t have to be more than that: for its main cause, the provision of KPIs to various kinds of organizational development projects, descriptive network metrics can be transformed and standardized, as shown with the collaboration score above. In our analysis we have tracked the success of our transformation and identified unused synergies between teams and can now focus on facilitating the collaboration between these specific business areas. More importantly, we can from now on evaluate our efforts in continuing our transformation with standardized KPIs. We have furthermore identified knowledge brokers and key players when it comes to uniting different parts of the organization in consulting projects.
At Kienbaum we are investing in the science of system simulation and setting up data teams to empower our clients in decision making processes throughout the entire HR value chain. We believe in the power of evidence based decision making – especially when it comes to people decisions.
For further information please contact Yannik Leusch (+49 30 88 01 98-49), People Analytics Lead.